5 Questions With . . . Fred Cohan on Moneyball, Biology
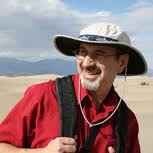
This issue, 5 Questions talks about the connections between the Moneyball and biology with Fredrick Cohan, professor of biology.
Q: Fred, you’ve been talking about how the data mining revolution in baseball, championed by the Michael Lewis book Moneyball and the recent movie of the same name starring Brad Pitt, can change science in general and biology, specifically. Really?
A: Absolutely! On the surface, Moneyball is the story of Billy Beane, the general manager of the Oakland A’s, who found a way to lead his poverty-stricken team to success against teams with many times the payroll of Oakland. But Moneyball is really about the thrill and triumph of data mining—how old data can be gleaned for meaning in ways that were never intended when the data were originally collected. Beane and his colleagues challenged the time-honored “holy trinity” of batting average, home runs, and runs batted in (RBIs) as the essence of a player’s offensive value. They were working off theories developed by Bill James, who posited in the 1970s that these traditional statistics, which everyone in the game knew provided the “truth” about a player’s value, were really imperfect measurements. James mined the data and developed other measurements, such as O.B.P. (on base percentage), which accounted for walks as well as hits, and slugging percentage, which accounted for the value of all extra base hits, not just home runs. What I particularly like about James’ approach is that he didn’t just replace one intuition with another. He let the game decide which stats really did the best job of predicting a team’s offensive output over the season. Beane decided to employ these and other data-mined statistics to build his team, despite great skepticism by his peers. The result was a string of playoff runs by a team with one of the lowest payrolls in baseball!
Q: Great for the As and baseball, but how can this help science?
A: As I see it, the baseball revolution has produced an “idiot’s guide” based on things one can learn, not through decades of baseball experience and intuition, but through application of general quantitative methods. Now that mountains of data and a capacity for analyzing them are also available in biology and other sciences, data can trump the intuition of experts and the “facts” that scientists have championed for years. For example, in biology, everyone “knows” what a species is: a group of organisms that can successfully interbreed, to produce viable and fertile offspring, and the origin of species is the origin of sexual isolation, the inability to interbreed. Biologists have long believed that species so defined are irreversibly separate evolutionary units, which can diverge without bound in their ecological characteristics.
Q: Can you provide a specific example?
A: In the case of my specialty, evolutionary microbiology, it is particularly important to be able to recognize all the ecologically distinct populations of bacteria, and we especially need to distinguish groups of close relatives that are dangerous from those that are not. To make the taxonomy more accessible, the field decades ago instituted a practice allowing anyone to use widely available molecular techniques to identify species, for example, a certain level of overall DNA sequence similarity. One popular universal criterion is to identify species as groups of organisms that are at least 99% similar in a particular gene. The problem is that, like the case of baseball where batting average, RBI, and home runs were used to supplement expert knowledge, nobody in microbiology tested whether the new molecular techniques actually came closer to solving the problem of recognizing the most closely related species. Unfortunately, microbiology’s current DNA-based idiot’s guide, as well as the expert-driven metabolic criteria that preceded it, has yielded species with extremely and unhelpfully broad dimensions. We can fix this in the same way that baseball improved its data analysis—by letting the game decide which stats best predict what we most want to know.
In biology, we can use the available data to let the bacteria tell us what DNA-sequence approach best identifies the bacteria that are significantly different in their habitats and ways of making a living. Two teams, including Martin Polz’s group at MIT and our group at Wesleyan and Montana State Universities, have developed computer algorithms for identifying groups of ecologically distinct bacteria specialized to different habitat types, within an officially recognized species. These algorithms reject the expert-based criteria for how much diversity should be placed within a species. Instead, they analyze the dynamics of bacterial evolution to let the bacteria tell us the DNA-sequence criterion that best demarcates ecologically distinct populations for a particular group of bacteria.
Q: You also think biologists could benefit from looking at the way baseball has ramped up the detail of recording its games, true?
A: In the past, recording of baseball data suffered from a lack of judgment and imagination about what might be useful for future analyses. For example, the record of a baseball game was traditionally based on a play-by-play approach that recorded the outcome of each at-bat; it was not until 1988 that a pitch-by-pitch account was reliably available. The pitch-by-pitch record has allowed analyses of such things as what constitutes abuse of a pitcher and how the probability of a successful at-bat changes with the count of balls and strikes.
Until recently, biology was equally short-sighted in its data collection, and this has created a problem for biologists who would like to analyze other scientists’ published data. For example, Lozupone and Knight recently analyzed published data on bacterial communities and were able to figure out that the most difficult evolutionary transition in the history of bacteria has been from adaptation from salty to non-salty habitats, and vice versa. However, because the original researchers did not record the actual salinity levels, Lozupone and Knight could not pinpoint the precise concentration of salinity that has been most difficult to cross in bacterial evolution.
Q: Are you saying that biologists and other scientists should collect as much data as possible at every opportunity?
A: The truth is that previous standards of data collection in biology were typically limited to what might be interesting for the experiment at hand, or perhaps for some future experiment in the same laboratory. Today, biologists are increasingly expected to anticipate likely uses by others of the data we gather and are taking pains to do so. But we will also be looking to more efforts – like baseball has done – to embrace “crowd intelligence.”
I recently discussed this with Hilmar Lapp, a database expert at the National Evolutionary Synthesis Center (NESCent). He said that it is too much to expect, in the case of biology, for one researcher to think to include all the observations worthy of recording for posterity. Accordingly, NESCent and other organizations have sponsored working groups to pool ideas and to propose standards and directions for biological data collection in some novel areas of inquiry—that is, to foster crowd intelligence. For example, the Genome Sequencing Consortium recently established standards for recording environmental data when genes and genomes are sampled; this presumably would have avoided the debacle of the missing salinity data that Lozupone and Knight encountered.
The Moneyball film opens with wisdom from Mickey Mantle: “It’s unbelievable how much you don’t know about the game you’ve been playing all your life.” Surely the same is true for many biologists pondering the organisms they have been studying all their lives. There is so much possibility for a data-driven explosion of understanding of games and creatures by explorers today and in the future. We owe these future explorers the most complete and useful record of life today that we can offer them.